If you haven’t already read the prior posts about our Product Intelligence Engine, then I recommend taking a look at them first:
Product Intelligence – A product-centric AI opportunity
With a focus on complete privacy protection, we have developed...
Read MoreExplore the impact of our Product Intelligence
In this post, we will explore the impact of our Product Intelligence on the quality of product recommendations at Hello Retail. The integration of our Product Intelligence Engine has resulted in a system that continuously improves, utilizing techniques such as image recognition and advanced semantic analysis. For example, if you sell wine, our engine can now identify its flavor profile and suggest similar wines your customers are likely to enjoy.
That sounds great!..
..but how do we use our proprietary Product Intelligence Engine for product recommendations?
Well, that is our main topic of the day. Hello Retail’s product recommendations solution, uses Product Intelligence in two ways: understand product relations and create better user personalization.
At Hello Retail, we have always offered access to an advanced recommendation builder, allowing you to tweak results to your own and your customers’ preferences: Do you need an upsell recommendation with two products matching the category of the product you are currently viewing? Would you like your customers to discover popular products similar in price to the item in their basket but from another product category?
Not an issue! You can do all of this and so much more.
To top it all off, we enhanced and fully enabled two of our product recommendation algorithms with the Product Intelligence Engine. The two new and improved algorithms are alternatives and bought together!
Alternatives
Most personalization vendors focus on a simplified approach by providing alternatives by showcasing products in the same category as the product you are looking at – however, that doesn’t work well if you don’t have well-structured category data. The other issue is that if you only compare products within the same category, you might end up with 100s of products, so which ones are closest to the product you are looking at?
Hello Retail’s alternatives algorithm utilizes diverse machine learning models to transform products into embedded vectors we can plot in our vector database. This transformation entails anything from language-agnostic text analysis to pricing and image analysis.
Using these techniques, we can easily find similar products. The benefit is that we perform a much more granular analysis and can identify how close products are to each other. Where other vendors use categories and might have 100s of products similar to each other, we can pick the few most alike, in combination with any additional strategy we want to apply.
See more details
Note: You can still benefit by having well-categorized products, especially for the filters for Search & Category listings – thereby, time invested in good data quality is time well spent.
Upsell
One major challenge for e-commerce businesses is that smaller language-specific webshops often lack sufficient sales and traffic information to provide relevant and compelling upsell recommendations.
Hello Retail overcomes this issue by analyzing purchase patterns across your stores to identify frequently bought together products. Our Product Intelligence Engine leverages the data from your primary shop to improve the performance of your smaller shops.
Another issue the Product Intelligence Engine addresses are frequent changes in product collections. If a new version of a Nike shoe is released this year and the SKU changes, it may seem like a new product to other vendors. However, Hello Retail recognizes the similarity between the old and new versions based on last year’s data patterns. Thus, providing you with accurate recommendations even when new versions of products are in stock. Doing so ensures that your product recommendations remain high-quality, regardless of changes in product collections.
Disclaimer: As a Hello Retail customer, you own all your data, and you can, at any time, request your data to be deleted. We guarantee that no personally identifiable or pricing information is shared across clients. Products in our system are stored as coordinates in a vector database. The vectors are mapped with other vectors to find, e.g., what has been bought together. All vector embeddings are hashed, which means that even the products are anonymous, as you cannot recover a product from its vector representation.
User Affinity
User Affinity is the way we understand store visitors and their preferences. We use Product Intelligence Engine to predict what they might be interested in, even if they have only viewed a single product. With little data, we can tell in advance what other items they may be likely to purchase.
Of course, we use user affinity in a way that ensures we don’t show the same products to all customers – where is the personalization in this?
One way of using personalization based on users’ preferences is in the upsell step. Doing so can ensure you first display two bought-together products of the visitor’s favorite brands before showing them other items bought together.
Let’s show you how
- Navigate to the recommendation strategy and apply a filter to the Brand that matches the UserBias (User Affinity). Remember to specify the number of products you want to return with the filter.
- Then add another Bought Together step and set the maximum number of products to 0.
This will fetch two upsell products from the user’s preferences, and then we will fill the rest with other good upsell suggestions.
What makes this unique is how we use the Product Intelligence Engine to infer other brands you are likely to purchase. If you are looking at a Nike shoe, we might know that you also have an affinity for brands like Adidas & Under Armour. So in the illustrated example, we will recommend upselling products from Adidas & Under Armour as well as the Nike product you viewed. This means that we, with little data, can expand the user profile based upon our understanding of product relations.
Of course, the inferred brands will not rank as high as your preferred brand, where the relationship is the strongest.
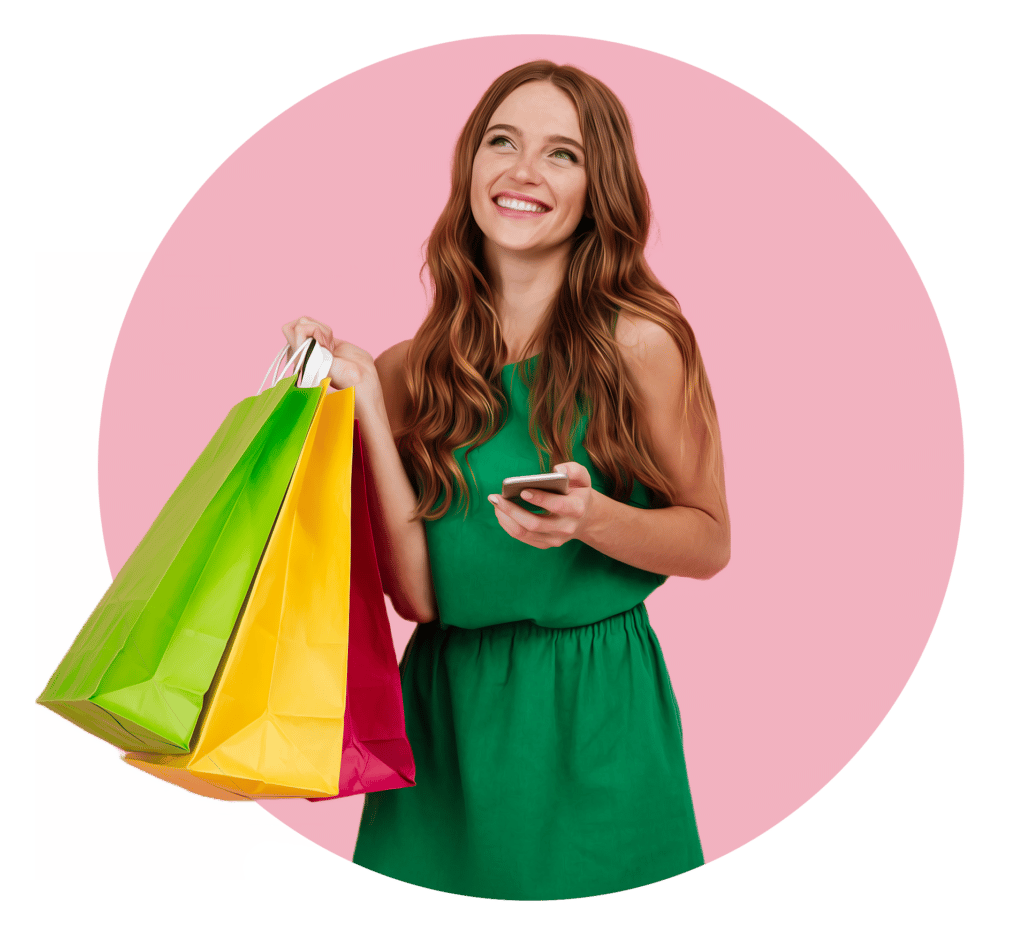
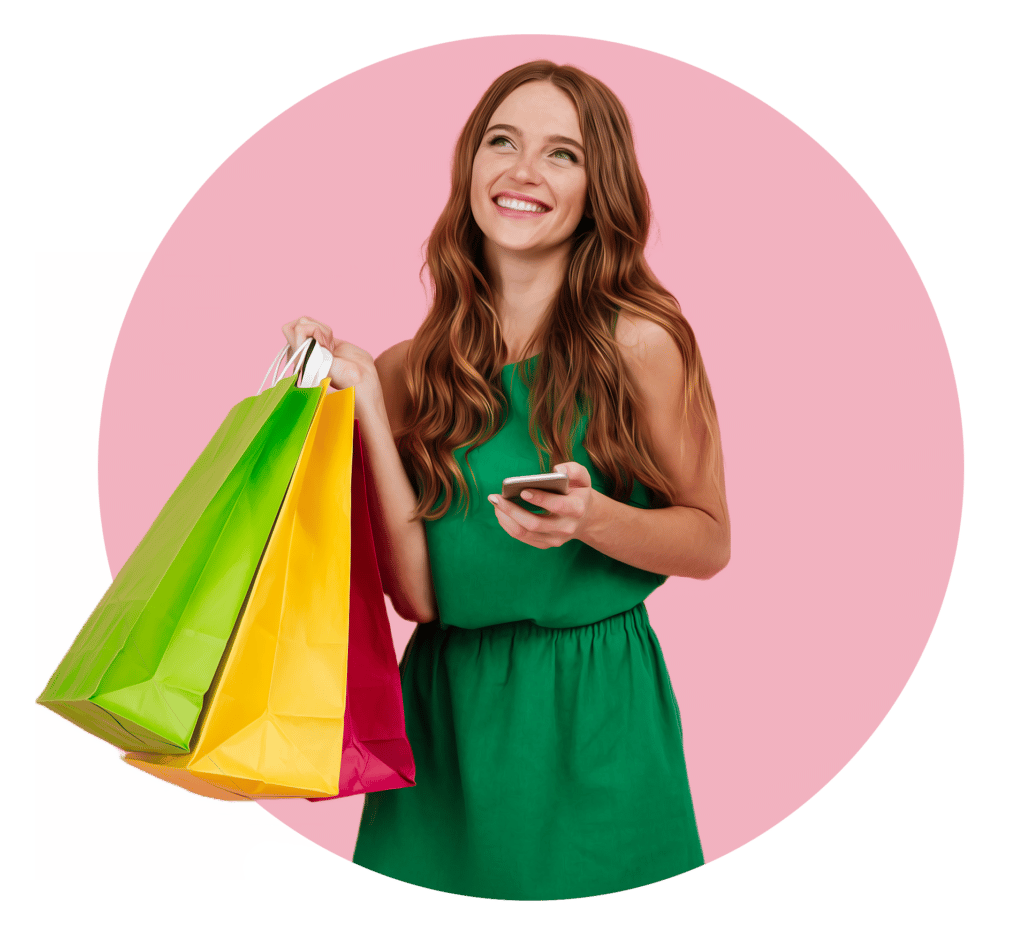
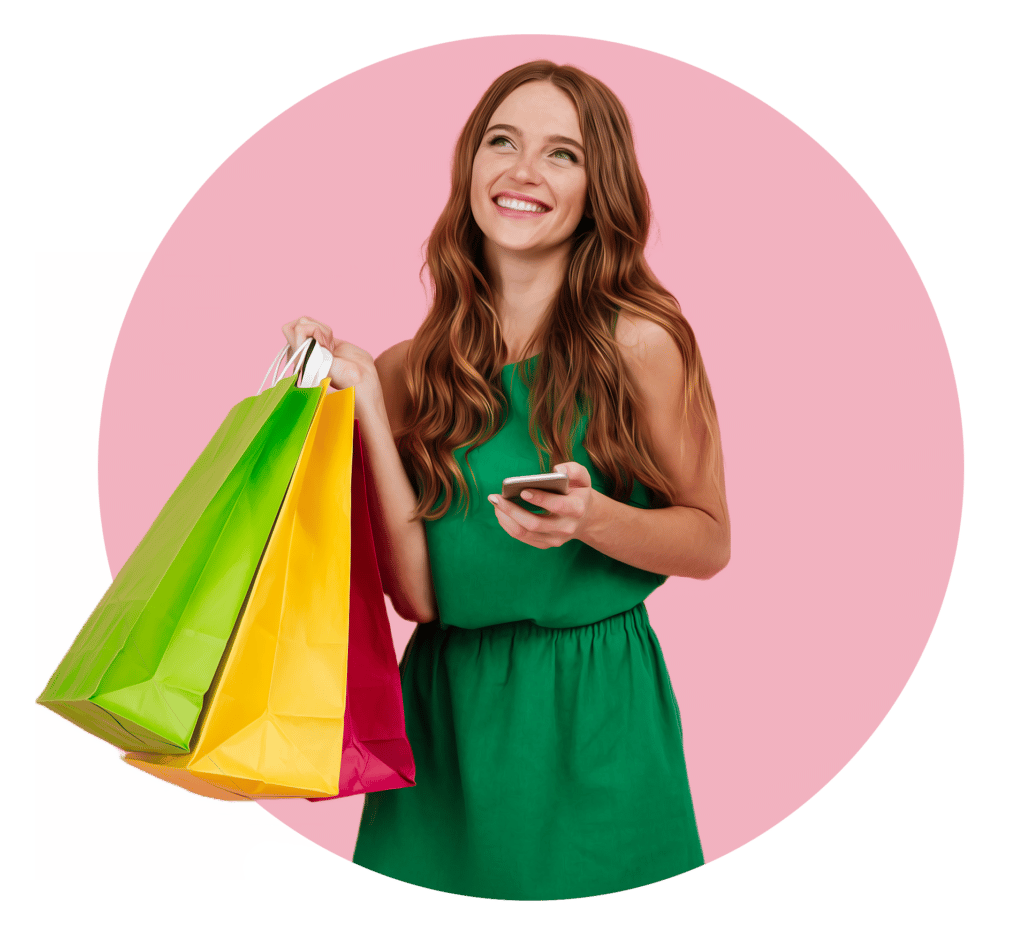
- BRAND: Nike
- Category: Shoes
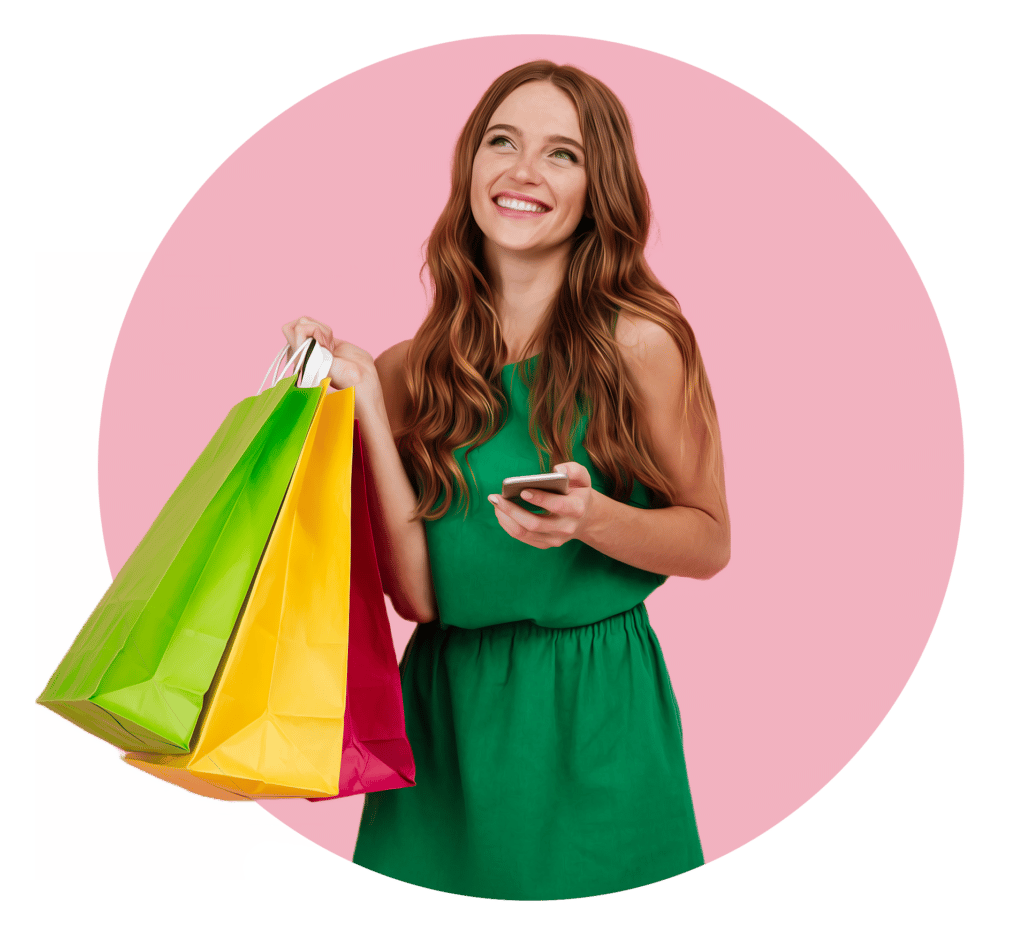
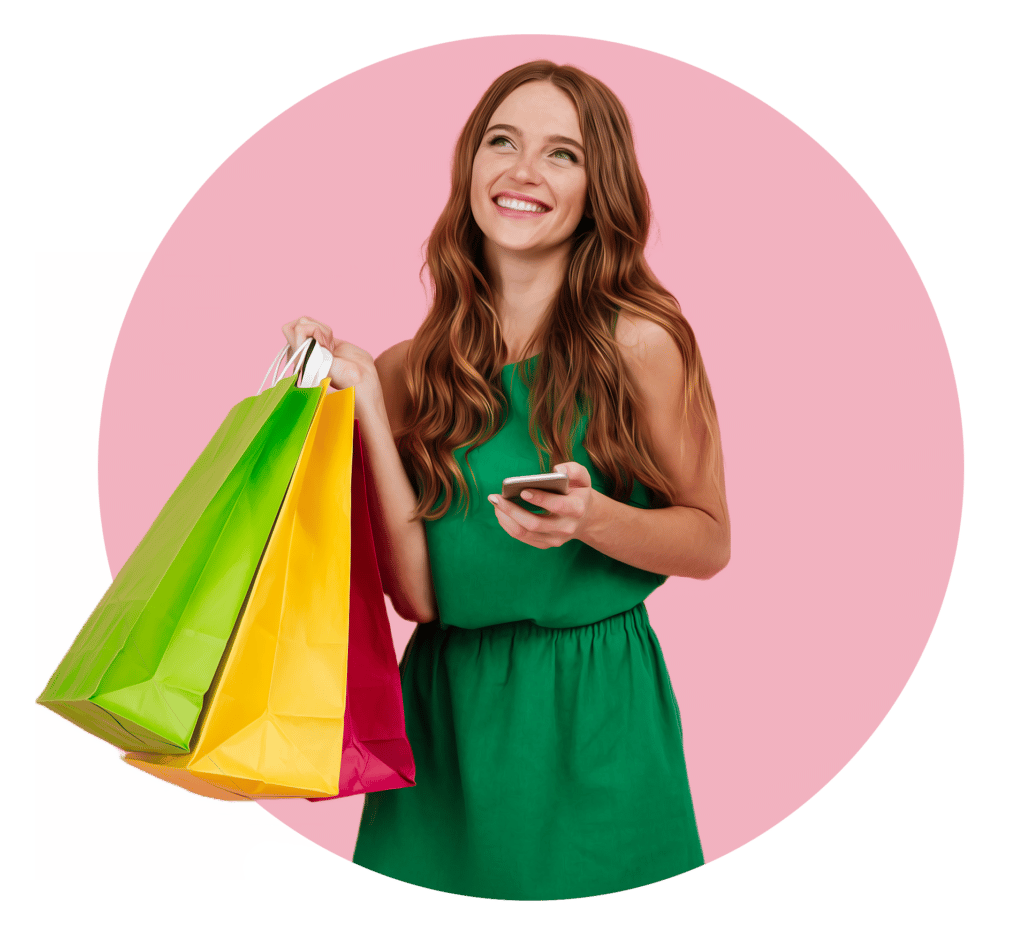
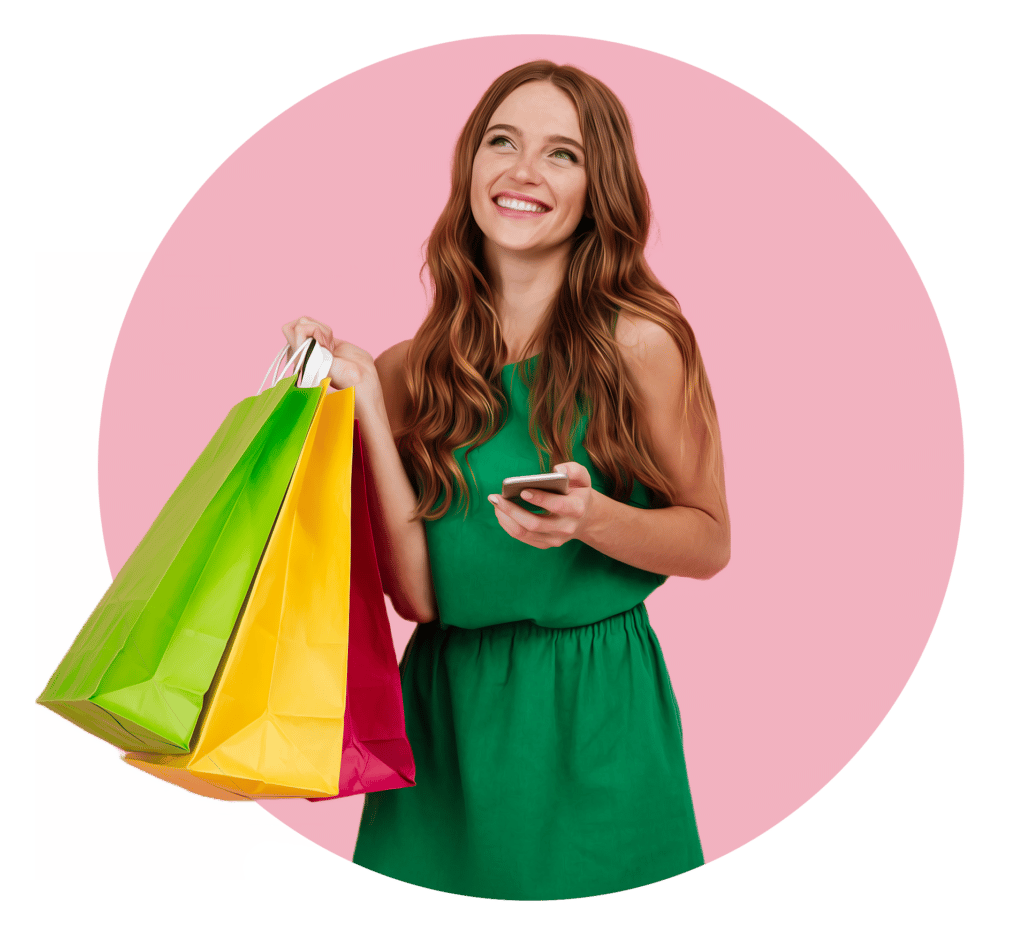
- BRAND: Nike, Adidas, Under Armour
- Category: Shoes, Shirts, Shorts
Show me the stats
We implemented split tests for some customers to verify and ensure better performance. The AB split tests were set up for the alternatives and upsell recommendations, with and without our Product Intelligence Engine, putting its capabilities to the test.
The majority of the stores which took part in the testing phase saw an improvement in CTR in the range of 35% to 117% with the Product Intelligence Engine.
All customers who participated in the tests saw a positive impact on soft and hard conversions*. The increase in soft conversions was between 8% and 41%, while hard conversions increased in the range of 2% to 99%(!). While we don’t assume all customers will see a 99% increase in the latter, we do have significant proof of the positive benefits our Product Intelligence Engine has on product recommendations.
*A soft conversion is when a customer clicks through the recommendations, but buys a product different than the one they clicked on. Hard conversions are considered those where a customer purchases the exact product they clicked on in the recommendation.
Coming up
Stay tuned for our next article, in which you will be able to read how we have utilized the solution to personalize each search result.